

Le système de stéganographie que nous proposons est basé sur l'utilisation de réseaux adverses génératifs. Nous proposons un algorithme d'insertion qui apprend automatiquement à insérer un message secrètement. Parmi les techniques existantes, nous nous concentrons sur l'approche du "jeu-à-3-joueurs". Les deux modules complémentaires permettent d'améliorer les performances de notre réseau.La deuxième contribution est l'application des techniques d'apprentissage profond à des fins stéganographiques i.e pour l'insertion. Parmi les amélioration possibles, nous avons évalué l'augmentation de la base de données d'entraînement, et l'utilisation d'un ensemble de CNN. De plus, Yedroudj-Net peut facilement être amélioré en ajoutant des compléments ou des modules bien connus. Comparé aux méthodes modernes de steganalyse basées sur l'apprentissage profond, Yedroudj-Net permet d'obtenir des résultats de détection performants, mais prend également moins de temps à converger, ce qui permet l'utilisation des bases d'apprentissage de grandes dimensions. Au cours des dernières années, plusieurs études ont montré que les réseaux de neurones convolutionnels peuvent atteindre des performances supérieures à celles des approches conventionnelles d'apprentissage machine.Le sujet de cette thèse traite des techniques d'apprentissage profond utilisées pour la stéganographie d'images et la stéganalyse dans le domaine spatial.La première contribution est un réseau de neurones convolutionnel rapide et efficace pour la stéganalyse, nommé Yedroudj-Net. Pendant une dizaine d'années, l'approche classique en stéganalyse a été d'utiliser un ensemble classifieur alimenté par des caractéristiques extraites "à la main". La stéganalyse d'image a elle pour objectif de détecter la présence d'un message caché en recherchant les artefacts présent dans l'image. La stéganographie d'image est l'art de la communication secrète dans le but d'échanger un message de manière furtive. For the steganalyzer we use Yedroudj-Net, this for its affordable size, and for the fact that its training does not require the use of any tricks that could increase the computational time.This second contribution defines a research direction, by giving first reflection elements while giving promising first results. The training of this steganographic system is conducted using three neural networks that compete against each other: the embedder, the extractor, and the steganalyzer. Our proposed steganography system is based on the use of generative adversarial networks.
#OUTGUESS SOFTWARE DOWNLOAD HOW TO#
Among the existing techniques, we focus on the 3-player game approach.We propose an embedding algorithm that automatically learns how to hide a message secretly. Among these add-ons, we have evaluated the data augmentation, and the the use of an ensemble of CNN Both increase our CNN performances.The second contribution is the application of deep learning techniques for steganography i.e the embedding.
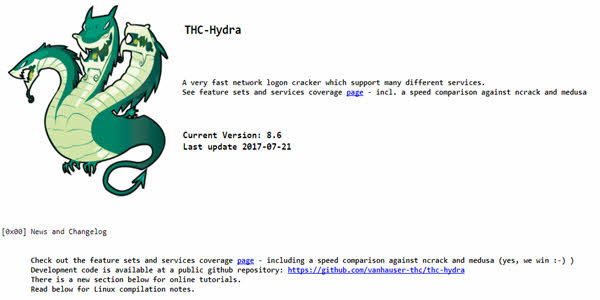
Moreover,Yedroudj-Net can easily be improved by using well known add-ons. Compared tomodern deep learning based steganalysis methods, Yedroudj-Net can achieve state-of-the-art detection results, but also takes less time to converge, allowing the use of a large training set. In recent years, studies have shown that well-designed convolutional neural networks (CNNs) can achieve superior performance compared to conventional machine-learning approaches.The subject of this thesis deals with the use of deep learning techniques for image steganography and steganalysis in the spatialdomain.The first contribution is a fast and very effective convolutional neural network for steganalysis, named Yedroudj-Net. For about ten years, the classic approach for steganalysis was to use an Ensemble Classifier fed by hand-crafted features. In the other hand, image steganalysis attempts to detect the presence of a hidden message by searching artefacts within an image.
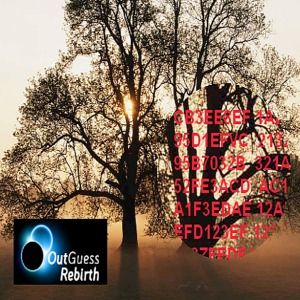
Image steganography is the art of secret communication in order to exchange a secret message.
